-
目次
- 0.1 Explore the Future of Technology with Quantum AI App – Revolutionizing Artificial Intelligence
- 0.2 Understanding the Fundamentals of Quantum Computing in AI
- 0.3 What Are Qubits and How Do They Differ from Classical Bits?
- 0.4 How Quantum Superposition Enhances Machine Learning Algorithms
- 0.5 Practical Applications of Quantum AI in Industry
- 0.6 Transforming Drug Discovery: Speeding Up Molecular Simulations
- 1 Understanding the Fundamentals of Quantum Computing in AI
- 2 Practical Applications of Quantum AI in Industry
Explore the Future of Technology with Quantum AI App – Revolutionizing Artificial Intelligence
-
Understanding the Fundamentals of Quantum Computing in AI
-
What Are Qubits and How Do They Differ from Classical Bits?
-
How Quantum Superposition Enhances Machine Learning Algorithms
-
Practical Applications of Quantum AI in Industry
-
Transforming Drug Discovery: Speeding Up Molecular Simulations
In recent years, advancements in computational capacity have transformed numerous fields, leading to breakthroughs that were once deemed impossible. One of the most fascinating developments is the emergence of quantum ai hybrid systems where complex algorithms intersect with subatomic phenomena. By leveraging these unique attributes, a new class of applications is on the brink of significantly enhancing how machines process information.
Businesses and researchers are already beginning to recognize the immense potential these systems offer. For instance, in optimization problems, enhanced processing capabilities allow for more accurate simulations and predictions, ultimately leading to faster decision-making. Companies in finance, logistics, and healthcare are reevaluating their operational frameworks to integrate these avant-garde solutions into their existing infrastructures.
The combination of high-dimensional data processing and advanced machine learning models enables a profound rethinking of how we approach problem-solving. Emphasizing real-time analytics, this integrated method fosters innovative solutions in various sectors, from personalized medicine to climate modeling. As organizations consider this paradigm shift, practical recommendations include investing in hybrid training frameworks and forming interdisciplinary teams to accelerate the deployment of these advanced models.
Understanding the Fundamentals of Quantum Computing in AI
Quantum computing represents a significant departure from classical systems, leveraging quantum bits or qubits to process information in ways previously unimaginable. Unlike binary states of 0 and 1 in traditional computing, qubits can exist in superposition, enabling calculations on multiple possibilities simultaneously.
Integration of this paradigm into machine learning can enhance data processing speeds and optimize complex algorithms. For instance, algorithms like Grover’s offer quadratic speedups for search problems, while Shor’s algorithm allows for rapid factorization, crucial for encryption tasks.
Moreover, quantum entanglement facilitates interconnected operations across qubits, leading to exponentially increased processing capabilities. This property can be harnessed to improve neural networks, enabling them to analyze patterns and correlations at a macroscopic scale.
When implementing quantum frameworks, it is essential to focus on error correction. Quantum systems are prone to decoherence, where external interference disrupts qubit states. Recent advancements in quantum error correction codes, such as the surface code, are instrumental in maintaining coherence and enhancing reliability.
Additionally, hybrid models combining classical and quantum components can offer practical solutions. By offloading specific computationally intensive tasks to quantum processors, systems can achieve better performance while relying on classical systems for regular processing needs.
Lastly, developing relevant skills in quantum programming languages, such as Qiskit or Cirq, is crucial for practitioners looking to tap into these advanced methodologies. Understanding quantum circuit design and gate operations will be pivotal as this domain evolves.
What Are Qubits and How Do They Differ from Classical Bits?
Bits, fundamental units in traditional computing systems, can represent either a 0 or a 1. In contrast, qubits possess unique properties that enable them to exist in multiple states simultaneously, thanks to principles of superposition and entanglement. This characteristic allows qubits to process information in a fundamentally different manner than classical bits.
Superposition enables a qubit to be in a state of both 0 and 1 at the same time, expanding computational capabilities exponentially. For example, while a classical bit can only represent a single state at any moment, a qubit can represent numerous combinations of states. This leads to significant improvements in processing power for specific complex problems.
Entanglement is another distinct feature of qubits. When qubits become entangled, the state of one qubit becomes linked to the state of another, irrespective of the distance separating them. This interconnectedness allows for more efficient computation and can lead to faster information transfer between qubits, enhancing overall system performance.
State Representation | 0 or 1 | 0, 1, or both simultaneously (superposition) |
Information Processing | Sequential | Parallel (due to superposition) |
Interdependence | None | Strong (entanglement) |
Computational Power | Limited | Exponential increase for specific tasks |
This fundamental difference positions qubits as powerful instruments in solving complex problems like optimization, cryptography, and simulating quantum systems. As research advances in this domain, understanding these distinctions becomes crucial for leveraging their capabilities effectively.
How Quantum Superposition Enhances Machine Learning Algorithms
Superposition is a fundamental principle in quantum mechanics that allows particles to exist in multiple states simultaneously. This concept can significantly improve machine learning models by enabling enhanced processing capabilities and solving complex problems more efficiently.
Here are several ways in which superposition benefits machine learning methodologies:
- Parallelism: Traditional algorithms often operate linearly, processing one state at a time. Superposition allows quantum systems to handle numerous possibilities concurrently, accelerating computation and reducing time complexity.
- Complex Probability Distributions: Quantum states can represent intricate probability distributions more naturally. This feature aids in better modeling of real-world situations, improving the accuracy of predictions.
- Feature Space Exploration: Quantum algorithms can traverse high-dimensional feature spaces more effectively, identifying patterns or correlations that classical methods might overlook.
To fully leverage this advantage in real-world applications, developers and researchers can consider the following strategies:
- Integrate Quantum Frameworks: Utilize existing quantum computing frameworks, such as Qiskit or Cirq, to develop hybrid models that combine classical and quantum processing capabilities.
- Focus on Low-Noise Environments: Ensure that quantum computations occur in low-noise conditions to maintain the integrity of superposition states and increase reliability of outcomes.
- Implement Error-Correction Protocols: Develop robust error-correction techniques to mitigate issues arising from decoherence and ensure that computations retain their expected quality.
By incorporating superposition principles into machine learning strategies, practitioners can enhance their algorithms’ efficiency and effectiveness, paving the way for breakthroughs in data processing and analysis.
Practical Applications of Quantum AI in Industry
Numerous sectors are capitalizing on advancements in quantum computing enhanced by intelligent algorithms, leading to transformative outcomes. One prominent domain is pharmaceuticals, where intricate molecular simulations enable researchers to predict drug interactions with unmatched accuracy. This reduces time spent in discovery, facilitating faster rollout of life-saving medications.
Finance stands as another critical area. Institutions are employing complex models powered by quantum-enhanced machine learning to analyze vast datasets. These techniques optimize trading strategies and risk assessments, promoting more informed decision-making and highlighting profitable opportunities that classical systems might overlook.
The supply chain management field benefits from real-time optimizations. By utilizing the capabilities of quantum computation, businesses can forecast demand and mitigate bottlenecks effectively. Enhanced algorithms streamline logistics, resulting in significant cost savings and improved service delivery.
In manufacturing, predictive maintenance applications are gaining traction. By analyzing equipment data patterns, organizations can anticipate failures before they disrupt operations. This proactive approach not only elevates productivity but also extends the lifespan of machinery, contributing to overall efficiency.
Telecommunications companies are also diving into this innovative realm. Leveraging quantum datasets, they enhance network security and optimize bandwidth allocation. Such advancements ensure seamless communication and safeguard sensitive information from cyber threats.
Retail is harnessing data-driven insights to refine customer experiences. Algorithms that integrate quantum methodologies analyze spending behaviors and preferences, helping retailers tailor personalized marketing strategies. Consequently, businesses can boost customer loyalty and drive sales growth.
In agriculture, precision farming approaches employing quantum insights optimize resource utilization. Farmers can better predict crop yields and simulate environmental impacts, leading to sustainable practices and increased productivity.
The intersection of quantum-enhanced systems and machine learning is just beginning to unlock potential across various industries. Utilizing these methodologies strategically can result in unparalleled advancements in efficiency, innovation, and responsiveness. Companies poised to adopt these approaches will significantly benefit from a competitive edge in their respective markets.
Transforming Drug Discovery: Speeding Up Molecular Simulations
Advancements in computational methodologies are reshaping pharmaceutical research. Utilizing high-performance algorithms, researchers are able to conduct molecular simulations with unprecedented speed and accuracy. By integrating quantum mechanics principles, these simulations provide deeper insights into molecular interactions, driving candidate selection efficiencies.
Conventional drug discovery processes often span several years, involving countless iterations of testing. However, leveraging sophisticated computational frameworks can reduce timeframes significantly. For instance, recent studies have indicated that employing advanced molecular dynamics simulations can accelerate identification of potential drug candidates by up to 30%.
Incorporating state-of-the-art machine learning techniques into simulation processes enhances predictive models. By training algorithms on vast datasets of molecular properties and behaviors, researchers can predict outcomes of complex interactions with significantly higher precision. This paradigm shift allows for prioritization of compounds with favorable characteristics, minimizing resources wasted on less promising options.
Collaboration between academia and industry plays a pivotal role in this transformation. Establishing partnerships enhances data sharing and fosters innovation pipelines. For example, companies that share anonymized simulation data can improve machine learning models while reducing duplicative efforts across institutions.
Additionally, real-time simulation capabilities enable iterative feedback loops in experimental designs, allowing researchers to adapt and refine their approaches dynamically. By simulating various scenarios and conditions, scientists can explore a broader spectrum of therapeutic possibilities rapidly, increasing the chances of discovering effective treatments.
Investment in cross-disciplinary teams, merging expertise in chemistry, computer science, and data analytics, is crucial. These teams can create robust frameworks that facilitate deeper investigation into complex biomolecular systems, ultimately yielding new drug candidates faster
In summary, by enhancing molecular simulations through advanced computational techniques and interdisciplinary collaboration, the drug discovery process can become more efficient, paving the way for innovative therapeutics that address unmet medical needs.
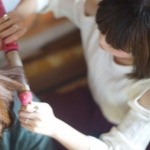
美人になりたい運営事務局
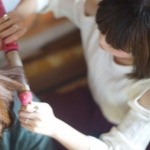